Migration services
We provide the most reliable tools to make sure you benefit from a smooth and successful migration that matches your needs and gives you a competitive edge.
Developers
Resources
Company
Company
ActiveEon is the new automation solution of choice. Learn about who we are and what we stand for.
Image Processing at Scale
Leverage cloud capacity and Activeeon elasticity
Cloud elasticity for Satellite Image Analysis
Activeeon awarded with Innovation prize at Paris Open Source Summit 2018
For image processing and more
Image processing is more and more common in cases such as:
- text recognition / optical character recognition (OCR)
- object detection with deep learning
- image segmentation with deep learning
- image classification with machine learning
- anomaly detection
- change detection
The processing of images require specific resources such as GPU or a big enough scale. At the CNES, satellites images needs to be processed to standardized for further processing. 7PB of images needs to transformed. The cloud capacity is consequently adapted to process such scale.
The CNES is leveraging Activeeon scheduling solution to distribute the workload over an elastic resource pool in the cloud.
Other use cases such as registration plate recognition, crop growth analysis, …. are projects requiring large scale compute power.
A solution built to scale
Scale to 20,000 CPU cores and distribute your application at scale
Setup an elastic policy based on the load / queue and pay for what you need only
Implement an architecture agnostic to the cloud provider and stay in control between your on-prem needs and cloud needs.
Some use cases
Image processing for satellite images analysis,
registration plate identification, crop analysis and more.
Distribution at scale and with precision
Automatically select the appropriate resource for your workflow
Schedule and automate
Build a strong basis for growth with template and standards. Give users advanced features with a user friendly interface.
Manage errors automatically
Handle errors natively and select the rule to adopt from a dropdown menu.
Optimize
Pay for what you need and nothing more
Any strategy: onprem, hybrid, multi-cloud
Embrace new resources with ease by adapting to your cloud strategy
End to end
Control govern and monitor processes from the launch to the resource
Integrates with every process
Languages and Connectors
Java,
Python,
Javascript,
Groovy,
Powershell,
Perl,
Control-M,
Dollar Universe,
SLURM,
PBS,
LSF,
SGE/OGE,
Matlab,
Scilab,
Language R,
Azure,
Amazon Web Services (AWS),
OpenStack,
VMware,
Docker,
Talend,
SAS,
Hibernate,
MySQL,
Postgres,
Oracle,
MariaDB,
…
For any situation
Move to open source solution for
Batch Processing,
Grid Computing,
Hybrid Clouds,
Java Workflow Engine,
Job Scheduler,
Open Source Workflow,
Parallel Computing,
Workflow Scheduling,
Workload Automation
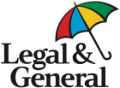
“Thanks to ProActive and additional optimizations we made along the way with Azure, the batch time which previously was taking us 18 hours, is now down to 5 hours, and by the end of the year, my hope and expectation is to go definitely below 4 hours and possibly less by having more grid hosts and more capacity.”
Guido Imperiale
Lead integration engineer at Legal and General
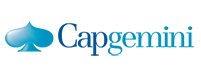
“Activeeon is the only solution capable to Schedule any Big Data Analytics, mono-threaded, multi-threaded, multi-core, parallel and distributed.”
CapGemini Lead Engineer for Home Office

“The ProActive product perfectly integrates into a hybrid multi-platform architecture. The usage of ProActive allowed us to have a trivialized approach to our processing infranstructure which includes Linux and Windows computers and GPU. This product helped us reduce operational costs by 10.”
Nicolas Pons
Head of InfoBioStat platform at MetaGenoPolis (INRA)